Binomial Effect Size Display: Let’s imagine a scenario where you are researching the COVID-19 vaccine. You gather data, analyze it, and find a statistically significant correlation between the disease and being cured from the vaccine. But wait while r (correlation) and phi values (for chi-square) provide valuable insights, they don’t reveal the full picture. How significant is the effect? Does it lead to actual improvements in patients? This is where the binomial effect size display (BESD) comes into the picture, serving as a powerful tool to connect statistical findings with real-world problems.
If you want to calculate Effect Size then here is the link: Effect Size Calculator
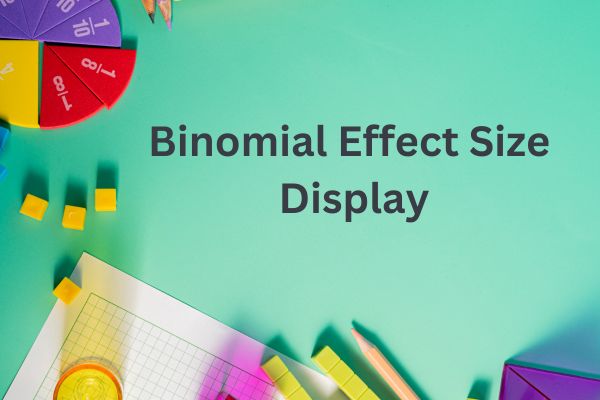
Before deep diving into the Binomial effect size display, let’s first understand what is Binomial effect size display.
Binomial Effect Size Display: Working, Use and Limitations
The binomial effect size display (BESD) concept was first introduced by Robert Rosenthal and Donald Rubin in the early 1980s, the BESD is a mechanism that is used to calculate the difference between the success rate of two groups in the experiment. The BESD is a favorable mechanism for researchers because it is simple and easy to demonstrate a measure of effect size.
How does binomial effect size display work?
Let’s take our previous example of COVID-19 vaccine effectiveness on patients.
Patients | cured | Not cured |
Vaccinated | 80% | 20% |
Not Vaccinated | 50% | 50% |
In the above table, let’s assume the research found a significant correlation between patients taking the COVID-19 vaccine and being cured from the disease. (It is represented by “cured” vs “not cured”).
We can see that,
- Among those vaccinated,80% are cured and 20% are not cured.
- Among those not vaccinated, only 50% are cured and 50% are not cured.
The above table suggests that the vaccine has a positive effect on cure. People who were vaccinated have 30% higher chances of being cured compared to those who are not vaccinated.
Why Use the BESD?
- Enhanced communication can be achieved by using BESD which interprets complicated statistics data into easily understandable data, this makes clear communication and easy understanding of research outcomes.
- Focus on practical significance a small statistically significant correlation might not be practically important, while a larger, non-significant correlation might still hold value.
- Improved interpretation can be achieved through BESD.
- Overcoming absolute terms unlike effect sizes, the BESD provides context for decision-making.
Limitations of the BESD
- The chosen BESD metric can influence the interpretation. Careful selection based on the research question and audience is crucial.
- BESDs can be sensitive to sample size. Smaller studies may produce less reliable effect size estimates. Replication and larger sample sizes are essential for confirming initial findings.
- Like any research method, BESDs can be affected by baffling variables that affect the outcome. Researchers should record these variables to ensure accurate results.
- Sometimes the data provided by BESD is difficult to understand by people who are unfamiliar with mathematics or statistics.
- BESD depends on data assumptions which sometimes may not always give true results.
Conclusion:
The BESD provides a precious tool for researchers seeking to communicate the practical importance of their findings. By interpreting correlation coefficients into success rates, the Binomial Effect Size Display bridges the gap between number data and real-world problems. Moreover, it is important to understand that binomial effect size display is not applicable for research areas due to its limitations.
Our Other Calculators